The integration of risk prediction models in clinical practice marks a transformative era in healthcare, where advanced algorithms offer valuable tools to assess and forecast specific outcomes. This paradigm shift contributes significantly to more informed decision-making, empowering healthcare professionals with enhanced insights into patient care. This essay embarks on a comprehensive exploration of risk prediction models in clinical practice, delving into their types, methodologies, significance, and providing illustrative examples.
Understanding Risk Prediction Models:
Risk prediction models serve as invaluable tools in clinical practice, harnessing algorithms to estimate the likelihood of specific outcomes. These models, designed to provide insights based on various factors and variables, play a crucial role in assisting healthcare professionals in assessing the probability of diseases, complications, or treatment responses for individual patients. The multifaceted nature of risk prediction models is evident in the three distinct levels: diagnostic, prognostic, and prescriptive models, each serving a unique purpose in shaping healthcare decision-making.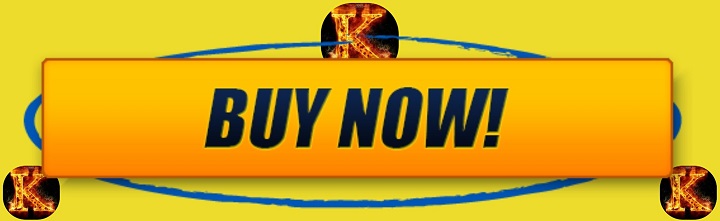
Types of Clinical Prediction Models:
-
Diagnostic Models:
- Diagnostic models focus on early disease identification, aiming to detect conditions in their initial stages when interventions are often more effective.
- Application: They are utilized in scenarios where early detection is crucial, such as cancer screenings or identifying risk factors for cardiovascular diseases.
- Significance: Diagnostic models contribute to timely and accurate diagnoses, enabling healthcare professionals to initiate prompt interventions and improve patient outcomes.
-
Prognostic Models:
- Prognostic models are designed to predict the likely outcomes and trajectories of diseases or health conditions over time.
- Application: These models are commonly employed in oncology, guiding treatment decisions based on the predicted progression of cancer, or in predicting the long-term outcomes of chronic conditions.
- Significance: Prognostic models assist healthcare providers in developing personalized care plans and optimizing resource allocation based on the expected course of a disease.
-
Prescriptive Models:
- Definition: Prescriptive models guide personalized treatment strategies, tailoring interventions based on individual patient characteristics and response patterns.
- Application: They are often used in areas like pharmacogenomics, where genetic information is utilized to predict an individual's response to specific medications.
- Significance: Prescriptive models contribute to precision medicine, ensuring that treatments are optimized for efficacy while minimizing adverse effects, leading to more personalized and effective healthcare.
-
Interconnected Roles:
- Complementary Nature: While each type has a distinct focus, these models often work in tandem. For example, a diagnostic model may identify a disease, a prognostic model predicts its progression, and a prescriptive model tailors the treatment plan accordingly.
- Holistic Patient Care: The integration of these models enables a comprehensive approach to patient care, from early identification and understanding the disease trajectory to tailoring personalized treatments for optimal outcomes.
-
Advancements in Machine Learning:
- Integration of Technology: Recent advancements in machine learning have significantly enhanced the capabilities of clinical prediction models.
- Data-Driven Precision: Machine learning algorithms can analyze large datasets, identifying intricate patterns and relationships that contribute to more accurate predictions.
- Examples: Risk prediction models in clinical practice benefit from machine learning algorithms, improving their ability to handle complex data and increasing the accuracy of predictions.
Read Also: Risk Modelling Methods
Methods of Risk Prediction:
The integration of risk prediction models into clinical practice represents a transformative leap in healthcare decision-making, providing essential tools for assessing and forecasting specific outcomes. Delving into the realm of risk prediction models unveils a sophisticated landscape of methodologies, each contributing to more informed decision-making. This exploration encompasses the types, methodologies, significance, and examples of these models, shedding light on their pivotal role in modern healthcare.-
Diverse Methodologies:
- Statistical Analyses: Risk prediction models often leverage statistical analyses to interpret data, quantify uncertainties, and establish associations between variables. Statistical methods provide a solid foundation for understanding the relationships that contribute to predicting outcomes.
- Machine Learning Algorithms: The advent of machine learning has ushered in a new era in risk prediction. Algorithms within machine learning, such as neural networks and decision trees, can discern intricate patterns in large datasets, allowing for more nuanced and accurate predictions.
- Epidemiological Principles: Rooted in epidemiology, risk prediction models draw on principles that explore the distribution and determinants of health-related events. This methodology aids in understanding how various factors contribute to the likelihood of specific outcomes.
-
Significance of Methodological Diversity:
- Comprehensive Understanding: The combination of statistical analyses, machine learning algorithms, and epidemiological principles provides a comprehensive understanding of risk factors and their interplay. This methodological diversity enhances the accuracy and reliability of predictions by capturing a broader spectrum of information.
- Adaptability to Varied Data Types: Different types of data require different analytical approaches. The diverse methodologies employed by risk prediction models allow them to adapt to various data types, whether structured clinical data, genetic information, or unstructured narratives from patient records.
-
Prominence of Machine Learning:
- Analyzing Vast Datasets: Machine learning, in particular, has gained prominence for its capacity to analyze vast datasets efficiently. This capability is crucial in the era of big data, where the sheer volume and complexity of information make traditional statistical approaches less feasible.
- Identifying Complex Patterns: Machine learning algorithms excel in identifying complex patterns and relationships within data. This ability contributes to the precision of risk prediction models, allowing them to uncover subtle nuances that may elude conventional statistical methods.
-
Examples of Methodological Integration:
- Risk Prediction in Cardiovascular Health: In cardiovascular risk prediction, statistical analyses may evaluate traditional risk factors like blood pressure and cholesterol levels. Machine learning algorithms can further analyze genetic data and lifestyle factors, providing a more holistic prediction.
- Cancer Risk Assessment: Epidemiological principles guide risk prediction models in cancer assessments, considering factors such as family history and environmental exposures. Machine learning algorithms enhance predictive accuracy by discerning subtle correlations within large datasets.
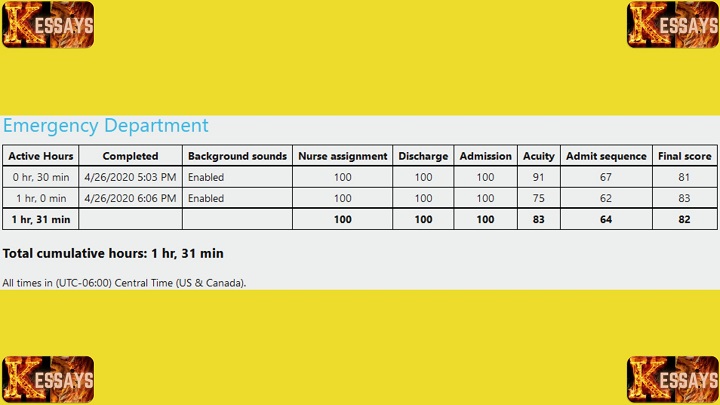
Three Predictive Models:
Risk prediction models in clinical practice operate on three primary levels, each serving distinct functions that collectively contribute to a holistic and patient-centric approach to healthcare. These three predictive models—diagnostic, prognostic, and prescriptive—play pivotal roles in prevention, diagnosis, and treatment, providing valuable insights to guide healthcare professionals in making informed decisions.-
Diagnostic Models:
- Diagnostic models are designed to identify diseases at their early stages, facilitating early intervention for optimal patient outcomes.
- Application: These models are particularly valuable for preventive care and early disease detection, enabling timely and effective interventions.
- Significance: By focusing on early identification, diagnostic models contribute to the prevention and timely management of diseases, reducing the overall burden on healthcare systems.
-
Prognostic Models:
- Prognostic models focus on predicting the likely outcomes and trajectories of diseases or health conditions over time.
- Application: In clinical settings, prognostic models guide healthcare professionals in understanding the potential course of a disease and its impact on a patient's health.
- Significance: Prognostic models provide crucial information for treatment planning, enabling personalized care based on the expected progression of a condition.
-
Prescriptive Models:
- Prescriptive models guide personalized treatment strategies, tailoring interventions based on individual patient characteristics and response patterns.
- Application: Employed in precision medicine, prescriptive models ensure that treatments are optimized for efficacy while minimizing adverse effects.
- Significance: By tailoring treatments to individual characteristics, prescriptive models contribute to more effective and personalized healthcare, aligning interventions with the unique needs of each patient.
Integration and Collaboration:
- Complementary Functions: Diagnostic, prognostic, and prescriptive models operate synergistically, addressing different aspects of patient care.
- Preventive Healthcare: Diagnostic models contribute to preventive healthcare by identifying risks early, while prognostic models offer insights into potential disease trajectories, enabling proactive management.
- Treatment Optimization: Prescriptive models enhance the treatment phase by tailoring interventions based on individual characteristics, optimizing treatment efficacy and minimizing adverse effects.
Patient-Centric Care:
- Comprehensive Patient Understanding: Together, these three models provide a comprehensive understanding of patients, covering aspects from disease identification to prognosis and personalized treatment.
- Informed Decision-Making: Healthcare professionals can make informed decisions by leveraging insights from diagnostic, prognostic, and prescriptive models, ensuring that interventions align with the unique needs and circumstances of each patient.
Read Also: Positive Predictive Value (PPV) in Diagnostic Testing
Examples of Risk Prediction Models: Illuminating Precision in Healthcare
Risk prediction models manifest their significance through practical applications, offering precise insights that guide healthcare interventions. Here, two compelling examples exemplify the real-world impact of such models: the Cardiovascular Risk Prediction Model and the Cancer Prognostic Model.-
Cardiovascular Risk Prediction Model:
- Factors Assessed: This model evaluates crucial factors, including age, blood pressure, cholesterol levels, and smoking status, to estimate the likelihood of cardiovascular events.
- Clinical Application: Clinicians utilize this model to implement preventive measures tailored to an individual's cardiovascular risk profile. For instance, if the model indicates high risk, interventions may include lifestyle modifications, medication, or other personalized strategies.
- Preventive Measures: The model's insights empower healthcare professionals to proactively address cardiovascular risk factors, preventing potential events and promoting long-term heart health.
- Personalized Interventions: Tailoring interventions based on the unique risk profile of each patient ensures a targeted and effective approach to cardiovascular care.
-
Cancer Prognostic Model:
- Factors Evaluated: Prognostic models in oncology delve into factors such as tumor stage, histology, and genetic markers to predict disease progression.
- Treatment Decision Guidance: The insights derived from this model play a pivotal role in guiding treatment decisions for cancer patients. By understanding the expected course of the disease, clinicians can tailor treatment plans to maximize efficacy and minimize adverse effects.
- Enhanced Patient Outcomes: The personalized approach facilitated by prognostic models contributes to improved patient outcomes. Avoiding unnecessary interventions and customizing treatments aligns with the unique characteristics of each patient, optimizing the overall success of cancer care.
- Precision in Oncology: In the rapidly advancing field of oncology, prognostic models exemplify the precision medicine paradigm, where treatments are not one-size-fits-all but rather tailored to individual patients based on their specific prognostic factors.
Significance of Examples:
- Tailoring Healthcare Interventions: Both examples showcase the ability of risk prediction models to tailor healthcare interventions based on individual risk profiles.
- Preventive and Personalized Care: The Cardiovascular Risk Prediction Model emphasizes preventive measures, while the Cancer Prognostic Model highlights personalized treatment strategies, collectively embodying a holistic and patient-centric approach.
- Real-World Impact: These examples underscore the tangible impact of risk prediction models in clinical practice, translating complex data into actionable insights that significantly enhance patient care.
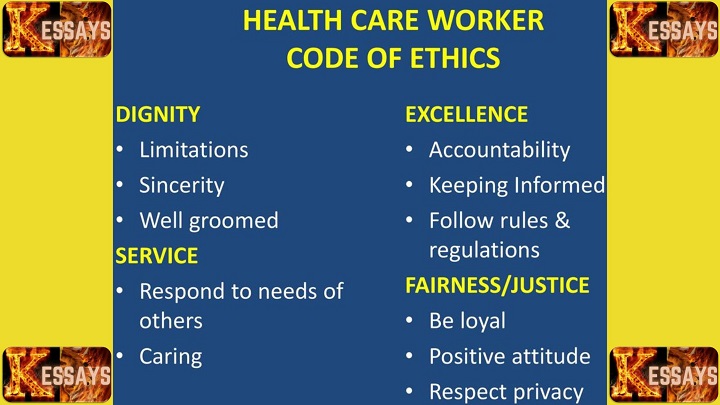
Role of Risk Prediction Models in Healthcare:
Risk prediction models stand as pivotal tools in healthcare, playing a crucial role in improving decision-making processes and elevating the precision of predictions. The integration of epidemiological principles and machine learning algorithms into these models signifies a transformative synergy, fostering a more accurate and insightful approach to healthcare interventions.-
Improving Decision-Making:
- Informed Decision-Making: Risk prediction models serve as decision support tools, enabling healthcare professionals to make more informed decisions by quantifying the likelihood of specific outcomes.
- Tailored Interventions: The insights provided by these models facilitate the implementation of tailored interventions. Healthcare professionals can customize treatment plans based on individual risk profiles, reducing unnecessary treatments and optimizing overall patient care.
- Preventive Measures: By quantifying risks accurately, risk prediction models contribute to the proactive implementation of preventive measures, ensuring early interventions that mitigate potential adverse outcomes.
-
Epidemiology and Machine Learning in Risk Prediction:
- Synergy of Disciplines: The collaborative integration of epidemiological principles and machine learning algorithms is foundational to the accuracy and effectiveness of risk prediction models.
- Incorporating Large Datasets: Machine learning's capacity to analyze vast datasets complements the principles of epidemiology, allowing for the incorporation of diverse and extensive data sources.
- Complex Relationships: The interdisciplinary approach embraces the complex relationships within healthcare data. Machine learning algorithms excel in identifying intricate patterns, contributing to a more nuanced understanding of risk factors.
- Enhanced Precision: The synergy elevates the precision of risk predictions, ensuring that models can decipher multifaceted data landscapes and provide healthcare professionals with insights that align with the complexities of patient health.
Significance of the Role:
- Individualized Patient Care: The role of risk prediction models in healthcare extends beyond statistical analysis. It encompasses the customization of patient care based on individual risk assessments, aligning interventions with unique patient characteristics.
- Resource Optimization: Informed decision-making facilitated by risk prediction models reduces the need for unnecessary treatments, optimizing the allocation of healthcare resources and minimizing the burden on healthcare systems.
- Proactive Healthcare:* The incorporation of preventive measures based on accurate risk assessments transforms healthcare into a proactive system, focusing on averting potential health issues before they escalate.
Read Also: Project Selection Methods: NPV, IRR, & Payback Period
Healthcare Essay Writing Services: Navigating the Complexity of Risk Prediction Models
As a student delving into the intricacies of risk prediction models in clinical practice, it's prudent to consider seeking guidance from reputable assignment help websites. Platforms such as kessays.com and peachyessays.com specialize in offering expert assistance, ensuring that you can craft well-researched essays that delve into the nuances of risk prediction models and their applications in healthcare. Additionally, online services like Kector.com, myassignmenthelp.com, and writersperhour.com can prove invaluable in enhancing your understanding of the subject and ultimately improving your academic performance.-
Expert Assistance for Complex Topics:
- Navigating Complexity: Risk prediction models in clinical practice can be complex, involving statistical analyses, machine learning algorithms, and epidemiological principles. Reputable assignment help websites provide expert assistance in navigating these complexities, ensuring that your essays are comprehensive and insightful.
- Well-Researched Essays: Crafting essays that delve into the types, methodologies, and real-world applications of risk prediction models requires thorough research. Professional writing services can aid in producing well-researched and well-structured essays that meet academic standards.
-
Diverse Platforms for Academic Support:
- Variety of Platforms: Consider exploring different platforms such as Kesity.com, myassignmenthelp.com, and writersperhour.com. These online services offer diverse perspectives and approaches to risk prediction models, enriching your understanding of the subject matter.
- Invaluable Learning Resource: These platforms not only assist with essay writing but also serve as valuable learning resources. Expertly crafted essays can serve as models for structuring your own work and comprehensively understanding the intricacies of risk prediction models.
-
Enhancing Academic Performance:
- Optimizing Understanding: Seeking assistance from these platforms is not just about completing assignments; it's about optimizing your understanding of risk prediction models. Expertly written essays can provide clarity on complex topics, contributing to a deeper comprehension of the subject matter.
- Improving Grades: By leveraging the expertise of these platforms, you can enhance the quality of your essays and, subsequently, improve your overall academic performance in the realm of risk prediction models.
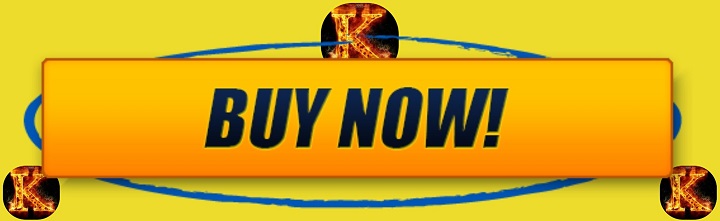
Comments are closed!